AI is everywhere these days due to its problem-solving capabilities at scale. The data-backed algorithms behind AI solutions also help reduce human error costs. We have seen AI in construction site safety assessments, chatbots, and accounting. But have you heard of AI in recruitment? These days, a growing number of companies have also turned to AI to boost recruitment efforts.
Despite its technological convenience, one rhetorical question lingers in the minds of many decision-makers: Can you trust AI in recruitment?
After all, the reliability and performance of AI technology depend on its training data. Outdated or biased databases could lead to a cycle of ineffective AI-based decisions. And when you factor those inefficiencies in recruiting, a core aspect of organizational success, they could undermine a company’s workforce, productivity, and bottom line.
So, employers need to understand the impact of AI in recruitment and address its impact on the delicate talent acquisition process.
How Does AI Work?
We should begin by visiting the technology’s origins to understand the current impact of AI in recruitment better. The earliest mention of modern AI dates back to 1955 at Dartmouth College when Professor John McCarthy coined the term for his Summer Research Project On Artificial Intelligence.
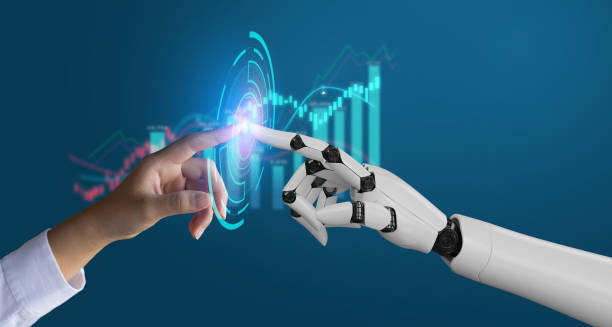
But, the discussion failed to meet expectations, ultimately delaying its progress. John’s concept eventually gained the attention of computer science specialists. Thus, leading to various applications and methods in the following decades.
The General Problem Solver (GPS) was one of the earliest AI solutions to apply its algorithms to problem-solving. It provided faster solutions for traditionally human-managed tasks.
Rapid advancements in AI technology eventually resulted in efficient language and image recognition capabilities. AI solutions have also become effective at producing quality inputs like text-to-image generation based on user prompts by learning human language and data patterns.
These led to multiple AI use cases, such as email generation, translations, and video transcriptions.
AI in Recruitment
The AI revolution eventually found its way into recruitment. Particularly, this is done by streamlining companies’ applicant tracking systems (ATS). AI’s consistency and predictive analytics functions enabled recruiters to monitor and track the most suitable candidates at scale based on specific criteria.
Predictive Analytics
Modern AI solutions enable employers to leverage historical workforce trends and other data sources (like trending workforce behaviors) to drive the most impactful recruitment campaigns. For example, predictive AI analytics can help recruiters check if a candidate is a good fit for the long term based on their experience and work history.
So, recruiters can use predictive analytics to narrow high-potential hires and optimize their talent acquisition budgets. Predictive analytics enable companies to take more calculated risks in hiring campaigns. This is done by forming decisions based on comprehensive data points, including past job performance, social media practices, and skill competencies.
Predictive analytics also enables employers and recruiters to improve hiring opportunities by identifying skill gaps in talent pools. Doing so enables companies to fill vacancies with talent with the right skills and experience to drive maximum productivity. Recruiting managers can apply these findings to fine-tune job descriptions (JDs) to attract top hires for a vacancy.
Interview Analytics
Interviews are critical to hiring processes, often considered the initial meeting between prospects and employers where it is important to leave a winning first impression. Workforce surveys show that one in three candidates reject a job offer due to negative candidate experiences, mostly due to poor communication.
So, employers must ensure they make the most of the interview process to learn more about candidates while leaving a good impression. Teams can implement AI solutions in interview analytics. This helps identify mistakes and red flags in an interview that could potentially lead to job rejections.
An advanced AI solution can provide real-time transcription and analytics of interviews that record candidate behavior and responses. These aids can provide interviewers with immediate cues/suggestions to engage candidates or critical insights for guiding future sessions. Companies could also use AI to update candidate personas based on the latest data.
For instance, AI-powered interview analytics can help companies create targeted questions based on compiled interviewee observations. One primary benefit of AI in interviews involves the detection of inherent biases that may occur in the interview.
These subconscious biases could turn away top hires and reduce the quality of hires. Interview biases may include similarity bias, where interviews favor employees with similar personalities and common traits. Interview biases also includes conformity bias, where multiple interviewers conveniently agree on a popular decision without genuine consideration.
Diversity and Inclusion Compliance
Diversity, inclusion, equity, and belonging (DEIB) are significant in successful modern organizations. Studies show that companies with diverse teams benefit from different viewpoints, leading to innovation and progress.
According to reports, Organizations in the top quartile for ethnic diversity are 36% more likely to outperform competitors. Other notable observations include inclusive teams being 35% more productive than less diverse groups and inclusive teams making better decisions (based on project outcomes and revenue) 87% of the time.
AI can help boost your company’s DEIB efforts by identifying and eliminating inherent biases from your talent acquisition initiatives.
For instance, Ongig’s Text Analyzer enables you to efficiently create effective and inclusive JDs from scratch based on a job title. The AI-driven solution provides a detailed recommended template to help you optimize every section of the JD to attract highly qualified candidates regardless of background.
The Text Analyzer also helps your HR team edit existing JDs, vetting them based on language, consistency, and biases to optimize them for conversions. Text Analyzer applies constantly updated research on DEIB terms and industry best practices to present effective JDs that engage the people your organization needs.
Text Analyzer’s AI efficiency enables your hiring team to refine JDs at any scale without the risk of human oversights or errors. The platform could prove highly effective, especially if you need to fill multiple urgent roles quickly.
The Benefits of AI in Recruitment
AI ultimately provides recruiters and employers with access to critical data that empowers better recruitment decisions in optimizing human resources. Outsourcing repetitive tasks to AI frees up hiring managers to focus on creating better talent engagement programs.
For example, a small business recruiter typically spends 30 hours a week on administrative tasks, which could be invested in more value-added areas. These tasks include lengthy interview processes (which could be due partly to unstructured sessions and inefficient applicant screening practices for trimming the list of qualified candidates).
Inefficient recruitment also leads to higher costs per hire since your company would spend more resources, including a dip in HR productivity when securing talent. AI essentially offers a respite for human intelligence in coping with the necessary paperwork.
AI Eliminates Interview Bottlenecks
Solutions that use AI in recruitment provide a systematic stream of data for conducting quality interviews. These data-driven processes can eliminate common bottlenecks that affect recruiting efficiencies. An AI-supported scheduling solution coordinates appointments that prevent double booking and overlaps when managing multiple candidates.
These include solutions like MeetingBird, which unifies recruiter communication via a single touchpoint. The platform utilizes natural language processing (NLP) via AI to recognize a company’s scheduling preferences, expediting candidate invitations.
The system’s AI also automates follow-ups, including pre-meeting reminders and post-interview engagements for shortlisted candidates. Similarly, companies can implement AI to resolve other interview bottlenecks. These include guiding informed decision-making via detailed comparative analytics of CVs and populating candidate scorecards for quicker, more consistent hiring.
AI Optimizes Talent Pool Outreach
Deloitte reported that 95% of CEOs consider diversity, equity, and inclusion a “personal strategic priority.” AI in recruitment can help companies advance these goals at the foundation of their company culture. Specifically, AI in recruitment provides an objective and time-effective solution to eliminating hiring biases.
These support companies in overcoming some of the most prevalent DEIB-based struggles that affect the modern workforce. For instance, Ongig’s Text Analyzer eliminates all bias in your job posting to attract hires solely based on relevant skills and past experiences.
The platform achieves this with reliable AI that syncs with the latest DEIB best practices, which check against potential prejudices, including gender, age, and ableism. Implementing the Text Analyzer’s AI-driven JD vetting function enables companies to navigate the talent gap in challenging job markets.
An AI-supported recruitment strategy can help target candidates from underrepresented groups for specific industries and specializations. For example, according to one industry survey, women fill only 28% of computing and mathematical roles. An AI-vetted approach to JDs can better engage women candidates by eliminating gender-coded words that could turn them away.
The Limitations of AI in Recruitment
As with any technology, there’s never a perfect “silver bullet solution.” Long-term use of AI has revealed flaws in its algorithms. Understanding these limitations is crucial and requires human intervention to address them strategically.
AI Hallucinations
Essentially, these hallucinations refer to mistakes or inaccuracies generated by an AI solution. Similar to human-experienced hallucinations, these occur when an AI makes up logic based on faulty data. When an AI system hallucinates, it predicts incorrect patterns according to flawed data inputs.
IBM defines AI hallucinations as faulty instances where the program “perceives patterns or objects that are nonexistent or imperceptible to human observers, creating outputs that are nonsensical or altogether inaccurate.”
Since AI systems rely on training models like the large learning model (LLM), data discrepancies within these structures affect the technology’s accuracy and performance. Kjell Carlsson, head of AI strategy at Domino Data Lab Inc., shared, “LLMs are notoriously unreliable, and they will occasionally fail even if you use them entirely the right way.”
So, relying on an AI system could lead to additional complications in recruitment rather than improving the flow.
The main causes of AI hallucination include:
- Incomplete training – This occurs when AI engineers omit critical parts of the learning process, resulting in faulty logic.
- Biased Data—AI engineers may integrate their inherent biases into AI learning, continuing the chain of exclusionary thought processes.
Examples of Hallucinations for AI in Recruitment
AI hallucinations can lead to significant misinformation and inaccuracies that reduce the efficiency of your hiring efforts. So, it is important to identify, prevent, and troubleshoot them at the first opportunity.
False Negatives
False negatives can occur due to AI hallucination, where a recruitment solution shortlists a qualified candidate who lacks the required credentials and experience for a role. In such cases, an AI system might misinterpret certain keywords or phrases in an individual’s CV to verify their suitability for a position.
For example, AI hallucination might identify the “director” in a person’s director of photography experience to qualify them for senior management roles in a film organization. In context, the Director of Photography is a technical position unrelated to company leadership. These AI inaccuracies could lead to costly and time-consuming fixes in managing job seekers at scale. .
Bias Amplification
AI hallucination could also reinforce existing human biases in the workplace. Hiring managers may implement recruitment systems with faulty AI (i.e., guided by flawed training data). These could tarnish your company’s reputation and severely limit your talent pool outreach.
For instance, your AI-supported recruitment programs may continue to overlook job applications from underrepresented groups despite their proven merits and qualifications for a role.
Assessing The Privacy Risks of AI in Recruitment
In addition to AI’s potential mistakes, it raises privacy concerns. AI uses various data sources to assess candidates. So, it’s vital to follow data privacy laws when using AI in hiring.
Informational Privacy Concerns
Keeping private information safe is a big challenge for businesses, especially in recruitment. It’s vital for companies to protect sensitive data when using it, including during the hiring process.
Data processing via AI could lead to legal complications in two ways. Firstly, AI’s continuous information collection could expose sensitive information from a data source. This includes personally identifiable information (PII), biometric information, and private financial data that fall under the protection of statutes like the California Data Protection Act (CDPA) and the Gramm-Leach-Bliley Act (GLBA).
Secondly, AI use involves the risk of predictive analytics. For instance, a sophisticated AI solution in recruitment could technically predict a candidate’s sensitive and confidential information based on learning models and algorithmic patterns. These may include traits like health statuses and political affiliations, which breach privacy practices and drive hiring biases.
Best Practices For Using AI in Recruitment
Your company can optimize the use of AI in recruitment by constantly monitoring and updating your software solutions. When in doubt, it is important to reach out to tech partners to clarify the technical aspects of their AI solution.
Trusted vendors should provide the assurance and guidelines to streamline your AI experience. This includes implementing robust AI training model testing procedures. Quality AI vendors should also perform continuous testing, updates, and integrations to meet the latest industry and privacy standards and guidelines.
Retain The Human Connection
AI tools are just like any other innovative solution. They might minimize human effort, but they still require human subject matter experts. The same concept applies to accountants replacing traditional paperwork with financial platforms and mathematicians replacing standard workings with calculators.
Human interaction is still vital for any business or organization. And AI simply streamlines results and performance with quality data. Your company needs human recruiters in the systematic and strategic use of artificial intelligence in talent acquisition.
Promote Explainable AI
Explainable AI (XAI) refers to AI solutions that help people understand how an AI process works and the ability to break technicalities down into their cause and effect. Software developer Violet Turri describes XAI as a “set of processes and methods that allows human users to comprehend and trust the results and output created by machine learning algorithms.”
XAI is critical for securing stakeholder trust and support when incorporating AI solutions into company practices, such as recruitment.
Rather than faithfully trusting their recruitment to AI automation, XAI gives users clarity on the steps behind AI-generated results. This would include a clear understanding of transparency in communication, handling sensitive candidate data, and qualifying the source of information utilized by an AI platform.
IBM highlights three major steps in XAI practices:
- Prediction accuracy: Comparing XAI outputs training data sets with programs like Local Interpretable Model-Agnostic Explanations (LIME) to monitor and manage data precision.
- Traceability: Structuring machine learning rules and regulations for easier tracking and management of system use.
- Decision Understanding: Providing users with basic AI training that raises awareness of technological functions and best use cases.
Maintain Open Communications With AI Vendors
To reduce AI mistakes in hiring, companies should talk regularly with tech vendors about issues they face. Clear communication ensures AI systems work correctly. So, vendors should explain templates, data sources, and training models when asked.
Establishing and revisiting AI expectations early in the collaboration minimizes the number of possible outcomes, which prevents inaccuracies and hallucinations. For instance, AI providers can implement a moderating process known as regularization. This penalizes a model for making extreme predictions that are usually off-tangent and inaccurate.
Prioritize AI Governance
Organizations can improve their control over AI with a structured approach to governance. These may include appointing a committee that oversees the frameworks and documentation of every AI implementation.
An AI governance committee could support hiring managers (and other departments) with the best AI practices for compliance while aligning with company principles and policies. One critical area in governance lies in outlining the goals and uses for AI. In recruitment, these could mean declaring the type of AI technology used for candidate sourcing and interview analytics.
Teams should then decide on the roles and duties assigned to each AI project. This can provide the transparency and accountability required to introduce and deploy AI solutions while minimizing the technology’s inherent risks.
Individuals managing governance within your organization should constantly revisit and evaluate AI systems to keep them updated with the latest industry and legal changes. Teams can ensure their systems remain efficient and compliant by referring to industry benchmarks like the OECD Principles on AI.
The OECD principles offer comprehensive coverage of AI optimization to inform policymakers while conforming to key values like explainability, transparency, and human-centered qualities (e.g., data protection and fairness). OECD also provides AI Incidents Monitor (AIM) documents containing detailed insights and AI hazards to optimize user experiences.
Conduct Routine Assessments
Your company could conduct routine internal audits to manage your hiring team’s use of AI more thoroughly. AI committee members could consider inviting external auditors and thought leaders for occasional assessments of company systems.
Industry specialists can analyze system data with thorough assessments, such as performance, data quality, and bias testing. These expert measures can provide critical insights for improving your AI in recruitment processes while aligning with ethical guidelines.
The Bottom Line
AI in recruitment has proven to be an efficient way to help streamline your hiring campaigns. The technology accelerates talent acquisition processes throughout the hiring life cycle. From optimizing JDs to engaging candidates throughout the ATS and enhancing interviews, AI can significantly improve the productivity of your recruitment campaigns.
But, it is important to note that a great variety of AI solutions are available. It is important to assess the reliability of each software before incorporating them into your organizational systems. Checking for user feedback and client testimonials and requesting trials can help you better gauge an AI’s efficiency and trustworthiness.
So, ultimately, can you trust AI in recruiting? Given AI’s cost-effective results, the answer leans toward a yes, but implementation should involve a gradual and cautious approach.
Your hiring team should always pair AI software with a human touch for the best recruitment campaigns. AI can oversee repetitive and tedious tasks (like scheduling interviews and generating quality JDs at scale) with proper guardrails. But, it is still down to your HR team’s acumen in interpreting AI data and selecting the best fit for your company’s lasting success.
Why I Wrote This:
Ongig is on a mission to replace biased and ineffective JDs with impactful alternatives that boost conversions. We achieve this through the limitless potential of AI technology via our Text Analyzer solution. With Text Analyzer, your hiring managers can scale recruitment practices with peace of mind, attracting top talent from diverse backgrounds. Want to see an example of AI in recruitment? Request a demo of Ongig’s Text Analyzer, today!
Shout-Outs:
- By Rockwell Anyoha, Harvard Graduate School of Arts And Sciences – The History of Artificial Intelligence
- By Max Roser, Our World In Data – The brief history of artificial intelligence: The world has changed fast – what might be next?
- LinkedIn Learning – Why is Diversity and Inclusion Important?
- By Steve Murphy, Forbes – AI In 2024: Beware Hallucinations, Pinpoint The Problem And Never Replace The Human Connection
- IBM – What Are AI Hallucinations?
- Stackshare – Calendly Vs Meetingbird
- By Kevin Newell, LinkedIn – AI Hallucinations in Staffing
- Deloitte – What rise in AI means for jobs, workers, organizations
- By Violet Turri, Carnegie Mellon University – What Is Explainable AI?
- LinkedIn – How Small Businesses Attract And Hire Top Talent
- OECD – How Can We Ensure That AI Benefits Society As a Whole?
- OECD AI Incidents Monitor (AIM)
- CIO, Women in tech statistics: The hard truths of an uphill battle
- By Jia Rizvi, Forbes – How AI Can Be Leveraged For Diversity And Inclusion
- By Brook Fischer, Homerun, – 12 types of hiring bias and how to avoid them